There could possibly be no greater conspiracy theory than the Simulation Hypothesis. It proposes that everything, with the exception of Nothing, is fake and essentially a manipulative alternative reality designed to fool our senses. Sounds like a dystopian episode of Black Mirror already? It doesn’t have to be.
The world of Synthetic Data is already all around us, and here to stay. And it is so much more than just deep fakes (a real and worrisome societal problem).
In this article, we want to impress upon you that synthetic data can be a force for good. And not just a force for good, that it can actually solve real problems with massive business outcomes that were previously thought to be impossible. We will then follow up with our observations and learnings from this market.
So let’s get right into it.
Synthetic data is a type of information that is artificially created or generated by computer programs or algorithms, instead of being collected from real-life sources. It is designed to mimic or resemble real data, but it is not derived from actual observations or measurements.
Today, most of us engage with AI-generated texts, videos, audio, and more already in our day-to-day lives.
You are more likely to have come across it in consumer use cases such as Chat GPT, text-to-image generation (we have all seen the stunning images created on Midjourney), gaming, social media, and communication.
You could try playing around with replica.ai, an AI companion — AI friend, mentor, and coach — personalized to users’ preferences and contexts that has clocked 10M+ in user base. It claims massive stickiness — with some users on the platform for 3–4 years now.
Another interesting company is hereafter.ai — Trained on people’s personal data, voice, information, etc in life, this app lets loved ones continue to talk to a virtual avatar (in the voice, style, and context of the person) after the person has passed away.
The technology has since matured — synthetic images, text, and speech generated have recently begun to be indistinguishable from human-generated content (see image below).

As the underlying technology has become mainstream and proven reliable for enterprise-grade usage, there has begun to be a B2C2B trend in the space with enterprises fast adopting and catching up to its possibilities.
Productivity-enhancement applications leveraging synthetic data are already all around us. Examples include Notion, Grammarly (for text), Photoroom, Runway ML (video production process enhancement and image editing tools), Synthesia (AI video creation for sales and marketing), Alta Voce (Voice-enhanced AI for customer support) and Replit (for Code).
More core applications of Synthetic data — targeted at R&D functions in businesses or machine learning applications — are slowly emerging.
Examples include training software for autonomously run vehicles and so many more.
Big Tech including of course Microsoft and OpenAI is pumping massive funding and resources into the synthetic data space today. Many new-age companies and open-source projects have emerged recently as well.
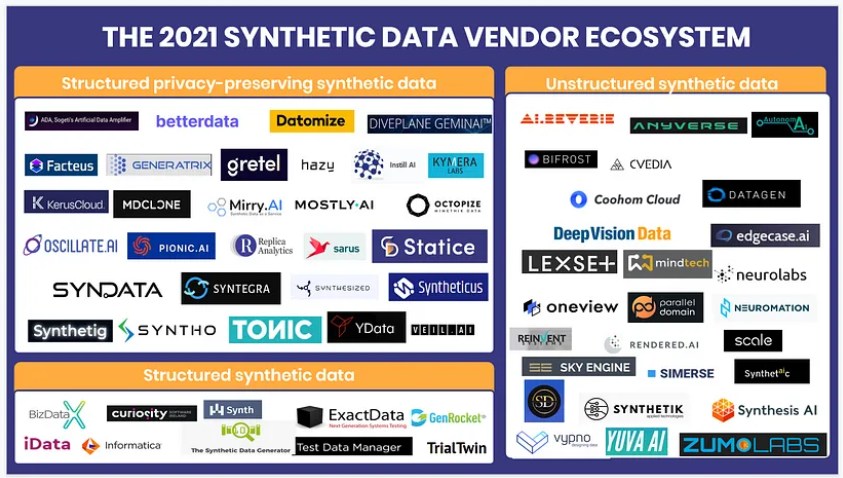
Companies in the Synthetic Data market can be largely divided into those generating structured data (largely tabular data) and those generating unstructured data (such as images, videos, etc).
Some companies in the Structured Synthetic Data space tend to be focused on privacy and cater to industries such as fintech or healthcare. The mechanisms of the creation of the synthetic data is optimized so as to avoid the reidentification of the original individual from it.
Synthetic Data and Why It Matters
The demand for Synthetic Data today arises from the convergence of multiple influential factors making it a timely and necessary topic of discussion. We lay out some of them in the following section.
Data-sharing and Data is a Bottleneck across industries
Limited data access is a barrier to data-driven research and development work in many industries today. If only for more data, better analytics could be done, and better ML models could be trained.
Teams, departments, and companies with access to data don’t share it with others for fear of private information leakage, trade secrets being read between the lines, or for lack of trust.
Also, more and more, complex AI workloads and analytics are being outsourced and transferred to vendors outside organizations. This further limits data sharing for the very use cases that the data needed to enable.
Some of this is also geopolitical — It is not acceptable today to share personal or sensitive data from one country to another today like it was a couple of years ago. Compliance and regulatory requirements like the GDPR, HIPAA, and laws protecting PII (Personally Identifiable Information) data of consumers are in effect in many parts of the world today. This means getting machine learning models that work well in local environments to transfer globally is a challenge.
Synthetic data is extremely useful in requirements like this as it solves for compliance and trust.
Examples include privacy-enhancing synthetic data for human genomic research.
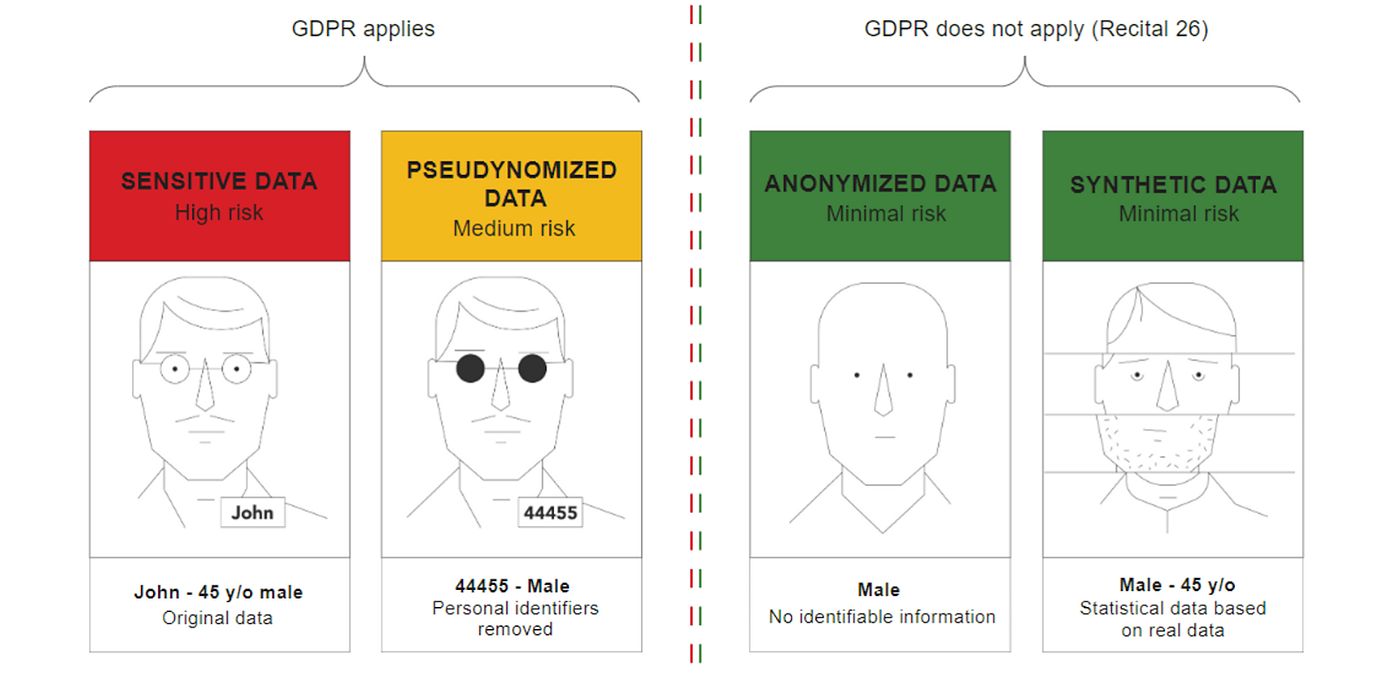
As shown above, GDPR and most regulations do not apply to both anonymized and synthetic data.
However, all kinds of ML models fare better on synthetic data than anonymized data making this the natural choice for most enterprises today.
Better training of Machine Learning Models
In 2021, Gartner predicted that 60% of data used for data and analytics by 2024 will be synthetic and not real. While 2024 is almost already here, directionally, there is reason to believe that the future is headed that way.
It’s a truism in the data science world that 80% of a data scientist’s work goes into data cleaning — labeling, annotating, structuring, and processing. This is something that synthetic data helps avoid (by nature it is labeled and structured), thus, saving costs and time. Buying into the bullish hypothesis that all models can be trained on synthetic data, the TAM (total addressable market) for synthetic data could be as large as the TAM for data itself.
Also, synthetic data is, by definition, ‘generated’. It is possible to generate data with parameters that a business might deem useful — for example, data that is sparse (of scenarios that occur infrequently) or difficult to obtain from the real world to train machine learning algorithms more thoroughly and on all kinds of edge case scenarios. This comes in handy in scenarios like financial fraud that occur in less than two percent of all transactions. Algorithms stress-tested and trained on these extreme scenarios tend to fare better in the real world.
Synthetic data is also a good way to tackle the problem of bias and fairness in AI models. This is made possible by intervening by injecting underrepresented data points into the input training datasets.
Additionally, there are other use cases where synthetic data for R&D or ML functions is useful. Some of them are as follows -
- When estimation or forecast models based on historical data no longer work
- When assumptions based on past experience fail
- When algorithms cannot reliably model all possible events due to gaps in real-world data sets
All three of the above were true during the pandemic of COVID-19, and seem to have helped with the adoption of synthetic data in multiple industries and for varied use cases.
Generative Adversarial Networks (GANs) power much of the synthetic data generation today
Some of the business problems that synthetic data solves for such as data sharing and regulatory compliance can also be tackled via alternative technologies like federated learning, data encryption, or statistical and mathematical modeling.
But they tend to be less sophisticated in their output quality or fidelity (a way to gauge the closeness of synthetic data to real-world data in all its structural characteristics), require expensive resources to handle, and don’t scale very well across all kinds of datasets and industries.
Generative Adversarial Networks (GANs) power much of the synthetic data generation today. At the core architecture level, GANs consist of two neural networks called a generator and a discriminator. The generator takes input data, adds random noise to it and generates artificial data. The goal of the training of GANs is to get the artificial data to be approved by the discriminator as if it were the original, input data. GANs rely on the discriminator being fooled into approving the artificial data created by the generator.
Essentially, Adversarial thinking — a faceoff and competition between the two neural networks — powers the generation of synthetic data.
Approval or rejection of the artificial data by the discriminator is a binary process inside GANs today. Imagine submitting a form into a portal and never knowing why it was rejected or not being provided pointed feedback on which specific field in the form needed a rework, and you were forced into iterating by trial-and-error multiple times to correct this. Today’s GANs work similarly between the generator and the discriminator and take a lot of compute cost to deliver synthetic data.
There are already early signs of research in this area that we are extremely excited and optimistic about. The technology for synthetic data generation and the use cases they can power are only going to get more powerful and mainstream in due time.
Our Observations
At Speciale Invest, we are interested and have been spending time in the data infrastructure space. Some of our observations on the Synthetic data market are as follows -
- The market for synthetic data is exploding. This is a real, pressing, and massive problem for many industries and will only grow. Most companies in this space are currently fairly early, indicating a potential opportunity in the space in the coming years.
- Synthetic generation of text, images, and even speech might be becoming a fairly solved problem today. Synthetic generation of 3D content, videos, or niche kinds of data with a sufficiently large Total Addressable Market (TAM) is a very interesting space.
- Synthetic Data is becoming a critical component of the Modern Data Stack. Most data scientists don’t sit down to think what data they currently don’t have but could better help business functions with, but this might not be for long.
- Given the resources OpenAI and Big Tech are pumping into this space, strong technology moats backed by academic research are necessary for companies building out another synthetic data company.
- Given how horizontal this technology is as a capability, for startups building out in the space, more verticalized and industry-specific workflows that are highly relevant to the users of these products will be a bonus.
- Given concerns about data privacy, security, and all the regulation surrounding this, a product in this market needs to have enterprise-grade features and be reliable.
If you are building a synthetic data company or are contributing to the Modern Data Stack in any way, we would like to hear from you and learn from your experiences. We want to brainstorm ideas, hear from you as to what is working in the market, and help you in any way we can. Please write to us at dhanush.ram@specialeinvest.com